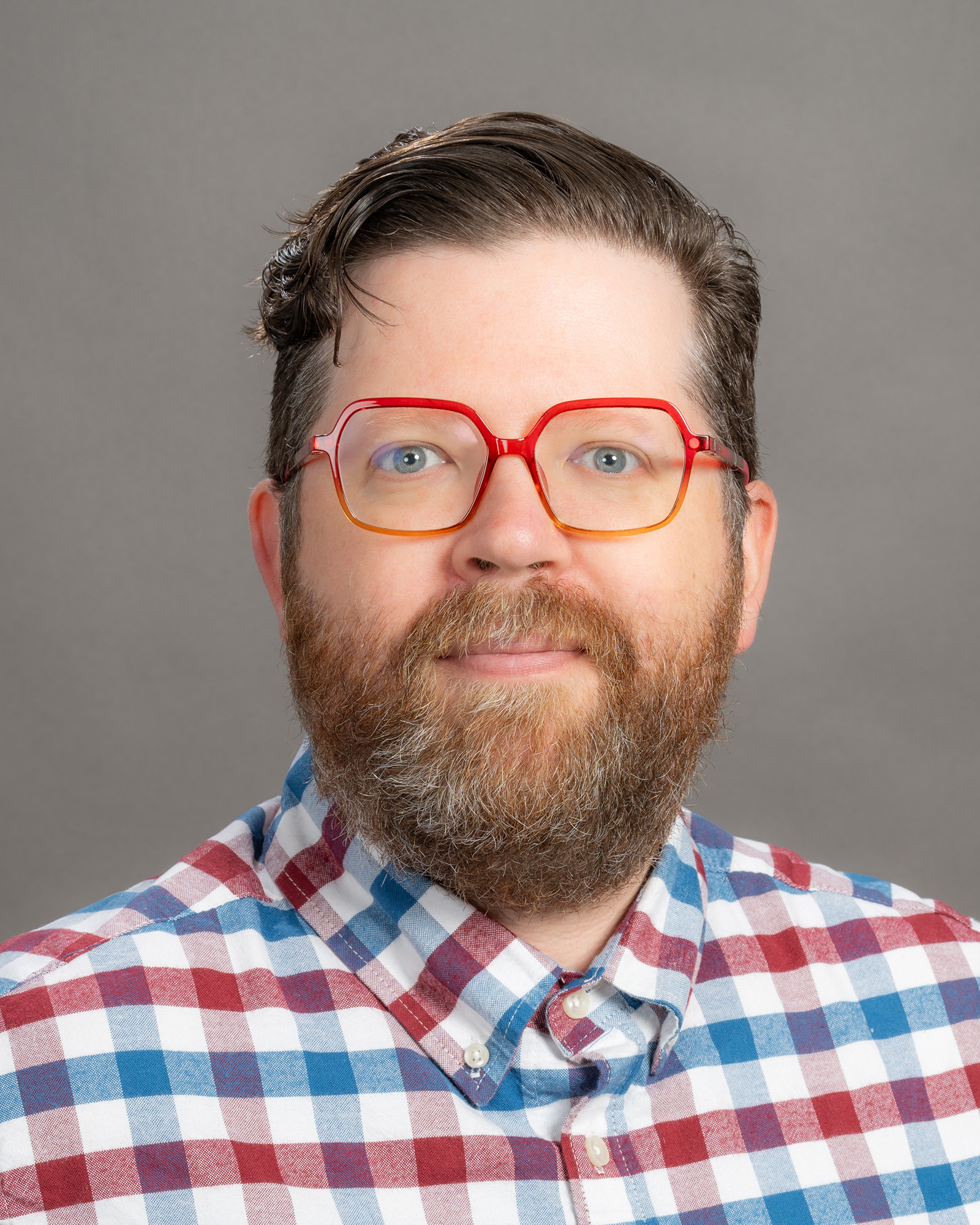
Associate Professor of Bioengineering
Associate Chair of Operations, Bioengineering
Member: Institute for Stem Cell and Regenerative Medicine
Member: Center for Cardiovascular Biology
Affiliate: eScience Institute
pmjboyle@uw.edu
Phone: (206) 685-1392
Office: Foege N310H (main campus); Brotman 311 (SLU)
Patrick M. Boyle
I lead the Cardiac Systems Simulation (CardSS) Lab in the Bioengineering department at the University of Washington (UW). Our lab uses computer models of the heart to cultivate new knowledge about cardiovascular disease. We use MRI scans from individual patients to power these complex “virtual heart” simulations. We use these powerful tools to discover underlying causes of disease or devise new treatment strategies that deliver better patient outcomes. We are predominantly interested in solving problems related to abnormalities in the heart’s electrical system (i.e., cardiac electrophysiology) but through our collaborations we are increasingly able to focus on biomechanics and fluid dynamics as well. Lastly, we are exploring the use of machine learning and artificial intelligence to help decipher complex problems in cardiovascular disease management.
- Predicting future health risks in patients with fibrotic remodeling of the left atrium (stroke, atrial fibrillation, etc.)
- Optimizing treatment of rhythm disorders via personalized simulations (catheter ablation, radiation therapy, etc.)
- Conceptualizing next-generation cardiac devices that harness new tech like optogenetics, harnessing light-based stimulation
- Investigating new applications of cardiomyocyte-like cells induced from patient-derived pluripotent stem cells
- Exploiting machine learning and AI to predict adverse cardiovascular events, with a particular emphasis on explainability
The Cardiac Systems Simulation (CardSS) Lab studies the human heart to better understand cardiovascular diseases, especially arrhythmias, and develop new treatments. Heart rhythm disorders are a growing concern, particularly among the elderly. Using computational heart models built from MRI scans, we aim to predict future complications like sudden cardiac arrest or stroke. We’re also using simulations as a means to explore regenerative medicine, using heart-like cells from stem cells to rejuvenate diseased hearts, and developing new biomedical devices, like light-based defibrillators. Our recent work uses AI and machine learning to analyze medical data (EKG, echocardiograms, MRIs) and improve long-term health outcomes, including in childhood cancer survivors. We’re also focused on advancing technology to make heart modeling faster and easier.
Our work is highly interdisciplinary, involving close collaboration with experts in high-performance computing, data science, biology, optics, medical imaging, and cardiology, particularly in treating arrhythmias. Our trainees are actively involved in translational research and participate in weekly clinical meetings with the UW Medicine cardiac electrophysiology team, where they learn alongside cardiology fellows.
An introductory lecture explaining some of the fundamentals of our lab’s research can be viewed at this link [https://drive.google.com/file/d/1gD_BMziTJmAJgq8NY3SZFDIScrgNa5I2/view]
Ph.D., Biomedical Engineering, University of Calgary, 2011
B.Sc. with Distinction, Internship program, Computer Engineering, University of Calgary, 2005
Assistant Research Professor, Department of Biomedical Engineering and Institute for Computational Medicine, Johns Hopkins University, 2015-18
Assistant Research Scientist, Institute for Computational Medicine, Johns Hopkins University, 2014-15
NSERC Postdoctoral Fellow, Computational Cardiology Lab, Institute for Computational Medicine, Johns Hopkins University, 2011-14
- American Heart Association / Additional Ventures Collaborative Sciences Award: “PULSE-SVA Network: Personalized Understanding from Linked Simulations & Electrophysiology in Single Ventricle Arrhythmia”, 2024-27
- Young Innovator in Cellular and Molecular Bioengineering, 2023, BMES
- University of Washington Department of Bioengineering Chair’s Award, 2023
- NIH R21-CA277746: “Machine Learning-Based Identification of Cardiomyopathy Risk in Childhood Cancer Survivors”, 2023-25
- NIH R01-HL158667: “Mechanistic Relationships Between Fibrosis, Fibrillation, and Stroke: Multi-Scale, Multi-Physics Simulations”, 2022-27
- Highest Scoring Abstract Award in the category of Digital Health, 2021, Heart Rhythm Society Annual Scientific Sessions
BIOEN484/584: Computational Modeling & Simulation of Bioelectricity (Autumn quarter)
Find more information on both courses on Dr. Boyle’s faculty website
Fibrosis as a Pathophysiological Nexus
- Macheret F, Bifulco SF, et al. Comparing Inducibility of Re-Entrant Arrhythmia in Patient-Specific Computational Models to Clinical Atrial Fibrillation Phenotypes. JACC Clin Electrophysiol. 2023 Oct;9(10):2149-2162. PMID: 37656099.
- Bifulco SF, et al. Computational modeling identifies embolic stroke of undetermined source patients with potential arrhythmic substrate. Elife. 2021 May 4;10:e64213. PMID: 33942719.
- Boyle PM, et al. Fibrosis, atrial fibrillation and stroke: clinical updates and emerging mechanistic models. Heart. 2021 Jan;107(2):99-105. PMID: 33097562.
- Boyle PM, Zghaib T. Computationally guided personalized targeted ablation of persistent atrial fibrillation. Nat Biomed Eng. 2019 Nov;3(11):870-879. PMID: 31427780.
Cardiac Optogenetics for Innovative Antiarrhythmic Therapy
- Ochs AR & Boyle PM. Optogenetic Modulation of Arrhythmia Triggers: Proof-of-Concept from Computational Modeling. Cell Mol Bioeng. 2023 Aug 24;16(4):243-259. PMID: 37810996.
- Ochs AR et al. Optogenetic Stimulation Using Anion Channelrhodopsin (GtACR1) Facilitates Termination of Reentrant Arrhythmias With Low Light Energy Requirements: A Computational Study. Front Physiol. 2021 Aug 30;12:718622. PMID: 34526912.
- Boyle PM, Yu J, et al. OptoGap is an optogenetics-enabled assay for quantification of cell-cell coupling in multicellular cardiac tissue. Sci Rep. 2021 Apr 29;11(1):9310. PMID: 33927252</>
Explainable Machine Learning and Artificial Intelligence
- Bifulco SF, et al. Explainable Machine Learning to Predict Anchored Reentry Substrate Created by Persistent Atrial Fibrillation Ablation in Computational Models. J Am Heart Assoc. 2023 Aug 15;12(16):e030500. PMID: 37581387.
- Sridhar AR, et al. Identifying risk of adverse outcomes in COVID-19 patients via artificial intelligence-powered analysis of 12-lead intake electrocardiogram. Cardiovasc Digit Health J. 2022 Apr;3(2):62-74. PMID: 35005676.
Understanding Arrhythmogenic Side Effects in Regenerative Medicine
- Gibbs CE, et al. Graft-host coupling changes can lead to engraftment arrhythmia: a computational study. J Physiol. 2023 Jul;601(13):2733-2749. PMID: 37014103.
Justice, Equity, Diversity, and Inclusion
- Linnes JC, et al. Framework for department-level accountability to diversify engineering. Nat Rev Bioeng. 2024;2:521-30. doi: 10.1038/s44222-024-00183-4.
- Cosgriff-Hernandez EM, et al. Equitable hiring strategies towards a diversified faculty. Nat Biomed Eng. 2023 Aug;7(8):961-968. PMID: 37580521.
In the News
UW Bioengineering faculty embrace AI tools to enhance teaching and learning
2025-03-21T07:09:14-07:00March 18th, 2025|
Engineering solutions for heart health
2025-02-24T07:37:49-08:00February 24th, 2025|
UW Bioengineering shines at the BMES 2024 annual meeting
2024-11-12T10:43:29-08:00November 12th, 2024|
Patrick Boyle joins collaborative effort to tackle arrhythmias in single ventricle heart disease
2024-09-13T06:03:08-07:00September 12th, 2024|
Faculty Promotions: Andre Berndt and Patrick Boyle
2024-06-12T05:35:48-07:00June 12th, 2024|
Patrick Boyle and Princess Imoukhuede receive 2024 ITHS Awards
2024-05-01T11:27:28-07:00January 9th, 2024|