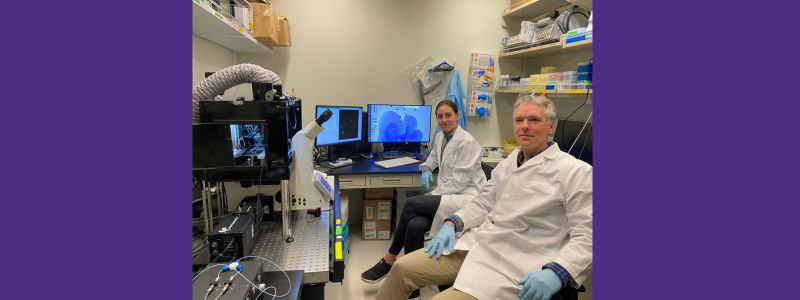
Andre Berndt, UW Bioengineering assistant professor, and Sarah Wait, PhD candidate in molecular engineering, in the Berndt lab.
Researchers with the University of Washington have discovered that machine learning, which is a branch of artificial intelligence (AI), can be utilized to solve complex protein engineering problems. By optimizing special proteins called Genetically Encoded Fluorescent Indicators (GEFIs) the proteins act like sensors inside living organisms, allowing researchers to visualize chemicals or signaling molecules in real-time. GEFIs work by attaching a part that lights up to a part that binds to specific molecules. When the sensor binds to its target molecule, it changes color or brightness, giving scientists a way to track what’s happening inside cells or organisms.
In the field of neuroscience, GEFIs have become incredibly important tools. They help researchers study various vital substances like calcium, dopamine and others in the brain. However, to make these sensors work effectively, scientists need to continually fine-tune their design. They often have to adjust various aspects of the sensor such as how sensitive it is or how quickly it responds to changes in the environment. This process of tweaking and optimizing the sensors can be time-consuming and expensive.
In a paper published by Nature Computational Science, researchers in UW Bioengineering Assistant Professor Andre Berndt’s group used machine learning to predict how different mutations to a specific GEFI called GCaMP would affect its behavior. GCaMP is a popular sensor used in neuroscience research, as it tracks calcium in cells and reports on neuronal activity. The Berndt lab has tested thousands of novel variants of GCaMP to learn how different mutations could affect its performance.
We used machine learning to predict how well mutated proteins might work, then tested the most promising mutations in the lab. Using this approach, we were able to quickly identify new mutants of the fluorescent calcium indicator GCaMP that work even better, both in terms of how fast they react and how brightly they glow. – Sarah Wait
The research team was comprised of lead author Sarah Wait, a graduate student in the Berndt lab; senior author Andre Berndt; and Institute for Stem Cell & Regenerative Medicine faculty members Michael Regnier, also a Bioengineering professor, David Baker and Farid Moussavi-Harami.
“We used machine learning to predict how well mutated proteins might work, then tested the most promising mutations in the lab,” Wait says. “Using this approach, we were able to quickly identify new mutants of the fluorescent calcium indicator GCaMP that work even better, both in terms of how fast they react and how brightly they glow.”
By feeding this existing data into their AI system, the authors were able to teach the computer to predict how new mutations might affect GCaMP’s properties. They then tested a limited number of the predictions in the lab and found that the machine learning system was quite accurate. It could identify mutations that made the sensor respond faster or produce a stronger signal when activated.
Machine learning allowed the team to create algorithms to analyze over a thousand new versions of GCaMP that hadn’t been tested before. And in the process, they discovered some new versions of GCaMP that respond faster and give stronger signals compared to existing versions. The team discovered the eGCaMP2+ version which can detect changes in calcium levels better than the more advanced versions of GCaMP that have been tested before.
“This work shows the power of machine learning in solving highly complex problems such as protein engineering,” Berndt says. “The algorithms improved a widely used calcium sensor, optimized over 20 years through heavy resources and personnel commitments, in a matter of months by a single person.” By harnessing the power of computers to analyze complex data, researchers can make the development of these important scientific tools faster and more efficient and engineer more sensors.