Amy Orsborn, Clare Boothe Luce assistant professor of Electrical & Computer Engineering and Bioengineering at the UW, has received a $3.5 million R01 grant from the National Institutes of Health. The project will allow the Orsborn lab to build algorithms that use brain-decoder interactions to make brain-computer interfaces (BCIs) work more reliably and longer for people with movement disorders.
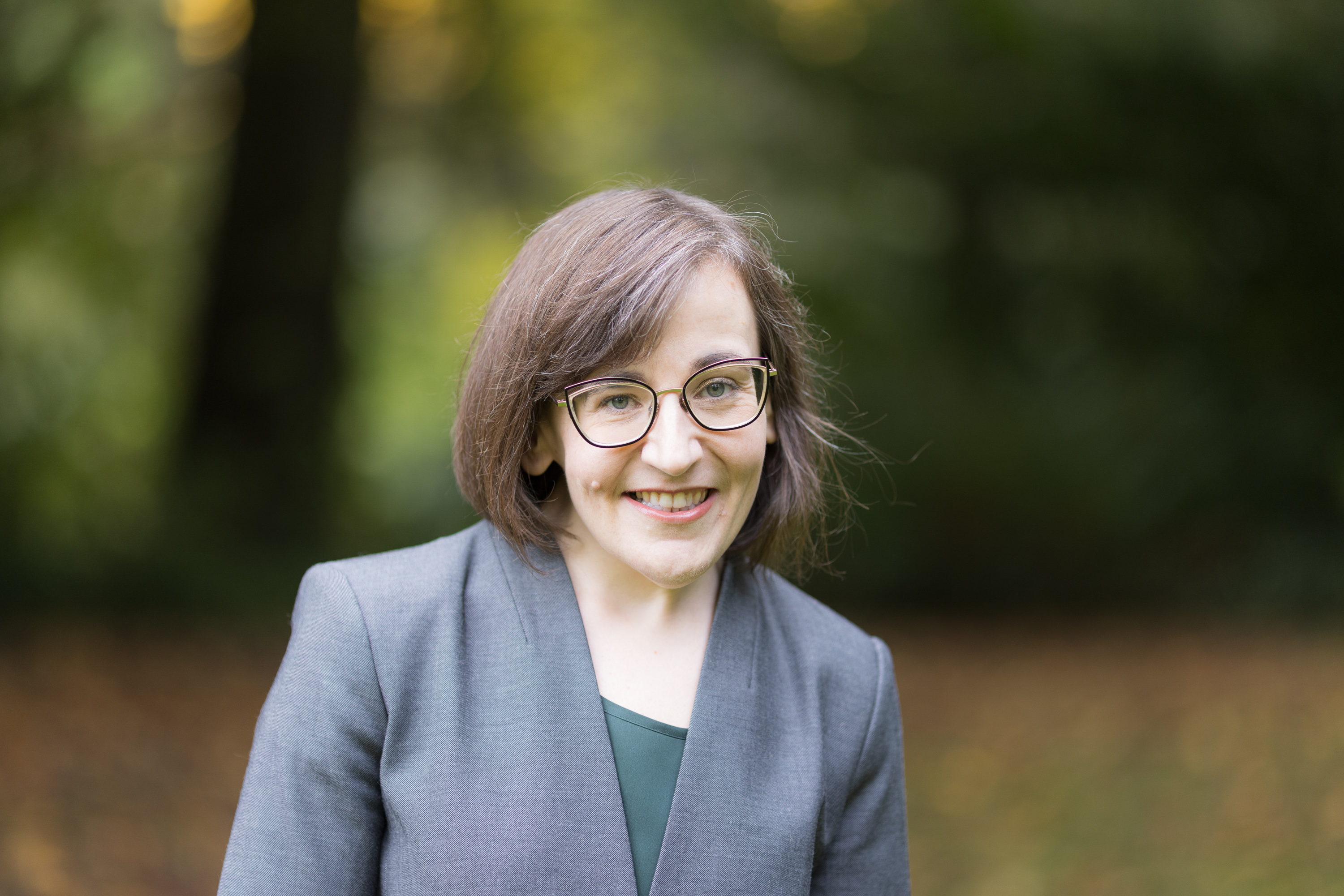
Interfacing technology with the nervous system holds tremendous potential to revolutionize how neurological disorders are treated. For example, BCIs can restore movement to people who have been paralyzed from a stroke, spinal cord injury or other trauma. BCIs use implanted sensors to measure the activity in someone’s brain as they think about moving and then translate these signals into movement commands for a device, like a computer or a prosthetic limb. These BCI use real-time machine learning to tap into remaining healthy parts of someone’s nervous system and reroute information past damage. This approach has allowed people unable to speak to type messages and people incapable of moving their limbs to control robotic prosthetics, purely with their thoughts.
Despite this promise, many challenges remain before BCIs will be widely available therapies. One critical challenge is assuring the devices work reliably when people use them for different tasks across their lifetime. Currently, BCIs are largely tested in laboratories with set tasks and for limited periods of time. But the goal will be for people to use a BCI for the many tasks they do every day, for years. Before BCIs can deliver this vision, researchers must overcome many obstacles.
It’s difficult to ensure BCIs work well for long periods of time in part because current sensors make it difficult to measure the same signals in a person’s brain every day. Changes in the measurements mean that researchers often must change the algorithms that interpret what movements someone intends to make based on the neural activity (the “decoder”). The Orsborn group and others have developed machine learning methods to smartly adapt algorithms to keep the BCI working well as measurements shift. But their recent work has revealed a potential problem with this approach, which motivates this new project.
The long-term goal of the project is to build algorithms that allow for changes in the brain and potentially even leverage them. Amy Orsborn
The root of the problem is that as an algorithm changes the decoder, the brain also changes. A person refines their tennis game over weeks, months and years of practice because their neurons are constantly refining their activity to control their movements. The same process happens as someone uses a BCI. Making the process more complicated, research has shown that how a device “decodes” the brain influences the brain’s learning. The Orsborn lab’s new findings reveal that interactions between the brain and decoder can cause major problems when current machine learning methods are used to adapt the algorithms. The existing approaches ignore changes in the brain, which might lead to unintended consequences such as the BCI no longer working due to how the brain adjusts over time.
“The long-term goal of the project is to build algorithms that allow for changes in the brain and potentially even leverage them,” Orsborn says. She predicts that researchers can use brain-decoder interactions to their benefit to make BCIs work more reliably for long periods of time as measurements change and when people perform different tasks. Achieving this goal first requires better understanding how the brain changes when someone practices with a BCI for an extended time, and how that is influenced by the decoder algorithm that is used.
The Orsborn lab will perform a series of studies to answer this question, with a particular focus on brain changes that will influence real-world BCI performance. Achieving their goal also requires that new algorithms take brain changes into consideration as they adapt the decoder. The team will test new frameworks they developed that allow BCI algorithms to weigh multiple goals simultaneously and compare them to existing methods. This will allow researchers to create next-generation BCIs that are aware of and can even shape learning in the brain to treat disorders.